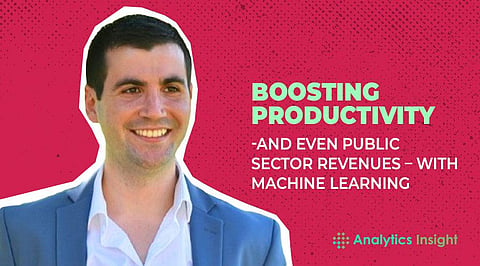
Did you know that countries around the world are losing significant amounts of tax revenue because they are unable to resolve or take on all the potential tax evasion cases in their jurisdiction? To make matters worse, many are not even aware of some of the tax evasion occurring. The stakes are quite high with money laundering, for example. According to Renolon, 90 percent of laundered money goes undetected, and the annual cost of this form of tax evasion is estimated to be over €823 billion in European countries and nearly $190 billion in the US each year. On top of that, the cases that analysts or investigators do work on are very time consuming, and it can become nearly impossible to follow the money trails to bring the cases to closure. But there is a better way: the use of machine learning (ML) is an opportunity for organizations like tax authorities to boost revenues by reducing time to intelligence, accelerating investigations and increasing case resolution rates.
Whether investigating money laundering or tax fraud, traditional methods of data analysis are time-consuming, inefficient and outdated. Analysts conducting financial investigations typically use a circular workflow: ask a question; sift through the data; surface insights; draw conclusions; and then repeat the process until the case is resolved. Using machine learning models, analysts gain valuable insights that cannot be extracted manually, especially with big data growing every day.
Machine learning leverages structured and unstructured data to perform pattern detection and generate classifications, predictions and more. The ML models generate sophisticated insights – not apparent by looking at the data itself – to help analysts make data-driven decisions more quickly for the cases they need to solve or mitigate. Over time, ML models also learn from existing and new data to provide even more data-driven decision intelligence.
New challenges are presented by both growth in the number of data sources and the increasing volume of data. While both can make an investigation more effective, they also make sorting through the data more challenging. Discerning which data is relevant to an investigation is simplified with ML's trend and pattern analysis. ML models can quickly and efficiently surface criminal patterns of behavior from the big data, providing analysts with sophisticated decision intelligence that helps to resolve cases. The reduced time to intelligence makes analysts more productive, so they can effectively investigate more deeply if required, are free to take on more cases, and can preserve revenue that would otherwise be lost.
The main objectives in money laundering investigations are to identify more illegitimate funds that are a major enabler for serious and organized crime. Successful combat against money laundering will not just diminish illicit activities but may also increase revenue for the state itself. As financial data sources evolve and become more fragmented, however, it is increasingly difficult for tax authorities to understand the full picture in money laundering investigations.
The financial source data may include bank records, financial assets, crypto transactions, invoices and more. From those disparate data sources, analysts need to gain insight on risk scoring, pattern anomalies, money trails and fraud detection – which is very difficult to accomplish manually. Using a data fusion system powered by ML, analysts can use ML models to see discrepancies between spend and receive. Specifically, the modeling uncovers stated activities of one source that do not match the declared activities of another source, revealing incoherence between declared receipts and stated activities. The combination of data fusion and ML enables the transformation of financial source data into unified entities and profiles, and then generates reports highlighting abnormal financial transactions.
For example, ML models can identify initial indications of a money laundering network by mapping hidden connections between suspicious entities and potential shell companies. From there, a system can automatically classify the involvement level of each entity using a risk score generated by an ML algorithm, and then identify potential suspects involved in fraudulent asset declarations. Analysts can also generate a timeline of suspects' actions and events for review and further analysis. The result: more illegitimate funds and transactions are identified, and analysts increase their case resolution rate, both of which increase revenue for a tax authority.
While there are many benefits of using ML, there are certainly some obstacles to overcome in increasing the use of ML in tax authorities and other public sector agencies. To some, machine learning may appear to be a "black box" – that is, analysts and others who approve the use of ML do not necessarily understand how and why the ML models generate the insights they do. The onus, then, is on companies that offer ML-driven decision intelligence systems to provide analysts with tools that make the process and results understandable.
At an organizational level, many countries and states have regulations that limit the use of machine learning models in investigations. For example, a case that is based primarily on ML insights may not be allowed. Analysts and investigators must show proof of human-based analysis and justification in combination with ML results to press charges against an individual or group for money laundering or tax fraud.
These obstacles are significant but not insurmountable. With time and better understanding, ML should prove to be an invaluable means of resolving cases.
ML brings new opportunities for public sector organizations to increase tax collection, clear more legitimate containers through customs, or resolve other revenue-generating bottlenecks. Using the decision intelligence generated by ML models enables these types of agencies to connect and make sense of all available data points even as data grows, increases in diversity and becomes more siloed. The bottom line: ML lets analysts and decision-makers see the big picture, quickly assess risks, conduct better investigations, and mitigate threats by leveraging the synergies between human and artificial intelligence.
More effective work, in less time, with more impactful results.
Tom Saltsberg, Product Manager, Cognyte
Tom Saltsberg is a product manager for Cognyte's decision intelligence platform. He has a strong background in web and open-source intelligence, as well as SIGINT. Tom holds a BS in computer science from the Hebrew University and is currently pursuing a master's degree in computer science at Reichman University.